Open Access
ARTICLE
Designing Bayesian Two-Sided Group Chain Sampling Plan for Gamma Prior Distribution
1 School of Quantitative of Sciences, Universiti Utara Malaysia, Sintok, 06010, Malaysia
2 Institute of Strategic Industrial Decision Modelling (ISIDM), Universiti Utara Malaysia, Sintok, 06010, Malaysia
* Corresponding Author: Nazrina Aziz. Email:
Computer Systems Science and Engineering 2023, 44(2), 1069-1079. https://doi.org/10.32604/csse.2023.022047
Received 26 July 2021; Accepted 27 August 2021; Issue published 15 June 2022
Abstract
Acceptance sampling is used to decide either the whole lot will be accepted or rejected, based on inspection of randomly sampled items from the same lot. As an alternative to traditional sampling plans, it is possible to use Bayesian approaches using previous knowledge on process variation. This study presents a Bayesian two-sided group chain sampling plan (BTSGChSP) by using various combinations of design parameters. In BTSGChSP, inspection is based on preceding as well as succeeding lots. Poisson function is used to derive the probability of lot acceptance based on defective and non-defective products. Gamma distribution is considered as a suitable prior for Poisson distribution. Four quality regions are found, namely: (i) quality decision region (QDR), (ii) probabilistic quality region (PQR), (iii) limiting quality region (LQR) and (iv) indifference quality region (IQR). Producer’s risk and consumer’s risk are considered to estimate the quality regions, where acceptable quality level (AQL) is associated with producer’s risk and limiting quality level (LQL) is associated with consumer’s risk. Moreover, AQL and LQL are used in the selection of design parameters for BTSGChSP. The values based on all possible combinations of design parameters for BTSGChSP are presented and inflection points’ values are found. The finding exposes that BTSGChSP is a better substitute for the existing plan for industrial practitioners.Keywords
Cite This Article
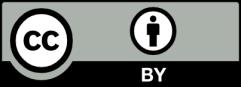
This work is licensed under a Creative Commons Attribution 4.0 International License , which permits unrestricted use, distribution, and reproduction in any medium, provided the original work is properly cited.