Open Access
ARTICLE
A Steganography Based on Optimal Multi-Threshold Block Labeling
1 Department of Information Engineering and Computer Science, Feng Chia University, Taichung, 407, Taiwan
2 Department of Electronic Engineering, National Quemoy University, Kinmen, 892, Taiwan
* Corresponding Author: Ji-Hwei Horng. Email:
Computer Systems Science and Engineering 2023, 44(1), 721-739. https://doi.org/10.32604/csse.2023.026046
Received 14 December 2021; Accepted 25 January 2022; Issue published 01 June 2022
Abstract
Hiding secret data in digital images is one of the major research fields in information security. Recently, reversible data hiding in encrypted images has attracted extensive attention due to the emergence of cloud services. This paper proposes a novel reversible data hiding method in encrypted images based on an optimal multi-threshold block labeling technique (OMTBL-RDHEI). In our scheme, the content owner encrypts the cover image with block permutation, pixel permutation, and stream cipher, which preserve the in-block correlation of pixel values. After uploading to the cloud service, the data hider applies the prediction error rearrangement (PER), the optimal threshold selection (OTS), and the multi-threshold labeling (MTL) methods to obtain a compressed version of the encrypted image and embed secret data into the vacated room. The receiver can extract the secret, restore the cover image, or do both according to his/her granted authority. The proposed MTL labels blocks of the encrypted image with a list of threshold values which is optimized with OTS based on the features of the current image. Experimental results show that labeling image blocks with the optimized threshold list can efficiently enlarge the amount of vacated room and thus improve the embedding capacity of an encrypted cover image. Security level of the proposed scheme is analyzed and the embedding capacity is compared with state-of-the-art schemes. Both are concluded with satisfactory performance.Keywords
Cite This Article
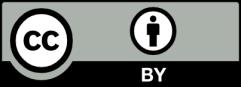
This work is licensed under a Creative Commons Attribution 4.0 International License , which permits unrestricted use, distribution, and reproduction in any medium, provided the original work is properly cited.