Open Access
ARTICLE
A Deep Learning Based Approach for Context-Aware Multi-Criteria Recommender Systems
Faculty of Information Technology, Quy Nhon University, Quy Nhon City, Vietnam
* Corresponding Author: Quang-Hung LE. Email:
Computer Systems Science and Engineering 2023, 44(1), 471-483. https://doi.org/10.32604/csse.2023.025897
Received 08 December 2021; Accepted 14 January 2022; Issue published 01 June 2022
Abstract
Recommender systems are similar to an information filtering system that helps identify items that best satisfy the users’ demands based on their preference profiles. Context-aware recommender systems (CARSs) and multi-criteria recommender systems (MCRSs) are extensions of traditional recommender systems. CARSs have integrated additional contextual information such as time, place, and so on for providing better recommendations. However, the majority of CARSs use ratings as a unique criterion for building communities. Meanwhile, MCRSs utilize user preferences in multiple criteria to better generate recommendations. Up to now, how to exploit context in MCRSs is still an open issue. This paper proposes a novel approach, which relies on deep learning for context-aware multi-criteria recommender systems. We apply deep neural network (DNN) models to predict the context-aware multi-criteria ratings and learn the aggregation function. We conduct experiments to evaluate the effect of this approach on the real-world dataset. A significant result is that our method outperforms other state-of-the-art methods for recommendation effectiveness.Keywords
Cite This Article
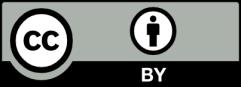