Open Access
ARTICLE
Detection of COVID-19 and Pneumonia Using Deep Convolutional Neural Network
Department of Electrical and Computer Engineering, North South University, Plot 15, Block B, Bashundhara, Dhaka, 1229, Bangladesh
* Corresponding Author: Sifat Momen. Email:
Computer Systems Science and Engineering 2023, 44(1), 519-534. https://doi.org/10.32604/csse.2023.025282
Received 18 November 2021; Accepted 19 January 2022; Issue published 01 June 2022
Abstract
COVID-19 has created a panic all around the globe. It is a contagious disease caused by Severe Acute Respiratory Syndrome Coronavirus 2 (SARS-CoV-2), originated from Wuhan in December 2019 and spread quickly all over the world. The healthcare sector of the world is facing great challenges tackling COVID cases. One of the problems many have witnessed is the misdiagnosis of COVID-19 cases with that of healthy and pneumonia cases. In this article, we propose a deep Convolutional Neural Network (CNN) based approach to detect COVID+ (i.e., patients with COVID-19), pneumonia and normal cases, from the chest X-ray images. COVID-19 detection from chest X-ray is suitable considering all aspects in comparison to Reverse Transcription Polymerase Chain Reaction (RT-PCR) and Computed Tomography (CT) scan. Several deep CNN models including VGG16, InceptionV3, DenseNet121, DenseNet201 and InceptionResNetV2 have been adopted in this proposed work. They have been trained individually to make particular predictions. Empirical results demonstrate that DenseNet201 provides overall better performance with accuracy, recall, F1-score and precision of 94.75%, 96%, 95% and 95% respectively. After careful comparison with results available in the literature, we have found to develop models with a higher reliability. All the studies were carried out using a publicly available chest X-ray (CXR) image data-set.Keywords
Cite This Article
M. Saiful Islam, S. Jyoti Das, M. Riajul Alam Khan, S. Momen and N. Mohammed, "Detection of covid-19 and pneumonia using deep convolutional neural network," Computer Systems Science and Engineering, vol. 44, no.1, pp. 519–534, 2023. https://doi.org/10.32604/csse.2023.025282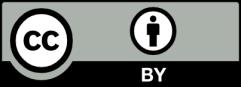