Open Access
ARTICLE
Deep Neural Network Based Detection and Segmentation of Ships for Maritime Surveillance
1 Department of Electronics and Tele-Communication Engineering, Jadavpur University, Kolkata, 700032, India
2 Department of Electronics and Communication Engineering, University of Engineering and Management, Kolkata, 700160, India
* Corresponding Author: Soumen Banerjee. Email:
Computer Systems Science and Engineering 2023, 44(1), 647-662. https://doi.org/10.32604/csse.2023.024997
Received 07 November 2021; Accepted 17 January 2022; Issue published 01 June 2022
Abstract
In recent years, computer vision finds wide applications in maritime surveillance with its sophisticated algorithms and advanced architecture. Automatic ship detection with computer vision techniques provide an efficient means to monitor as well as track ships in water bodies. Waterways being an important medium of transport require continuous monitoring for protection of national security. The remote sensing satellite images of ships in harbours and water bodies are the image data that aid the neural network models to localize ships and to facilitate early identification of possible threats at sea. This paper proposes a deep learning based model capable enough to classify between ships and no-ships as well as to localize ships in the original images using bounding box technique. Furthermore, classified ships are again segmented with deep learning based auto-encoder model. The proposed model, in terms of classification, provides successful results generating 99.5% and 99.2% validation and training accuracy respectively. The auto-encoder model also produces 85.1% and 84.2% validation and training accuracies. Moreover the IoU metric of the segmented images is found to be of 0.77 value. The experimental results reveal that the model is accurate and can be implemented for automatic ship detection in water bodies considering remote sensing satellite images as input to the computer vision system.Keywords
Cite This Article
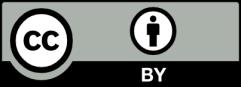