Open Access
ARTICLE
An Advanced Dynamic Scheduling for Achieving Optimal Resource Allocation
1 Department of Computer Science and Engineering, AAA College of Engineering and Technology, Sivakasi, Tamilnadu, India
2 Department of Information Technology, Mepco Schlenk Engineering College Sivakasi, Tamilnadu India
* Corresponding Author: R. Prabhu. Email:
Computer Systems Science and Engineering 2023, 44(1), 281-295. https://doi.org/10.32604/csse.2023.024339
Received 14 October 2021; Accepted 22 December 2021; Issue published 01 June 2022
Abstract
Cloud computing distributes task-parallel among the various resources. Applications with self-service supported and on-demand service have rapid growth. For these applications, cloud computing allocates the resources dynamically via the internet according to user requirements. Proper resource allocation is vital for fulfilling user requirements. In contrast, improper resource allocations result to load imbalance, which leads to severe service issues. The cloud resources implement internet-connected devices using the protocols for storing, communicating, and computations. The extensive needs and lack of optimal resource allocating scheme make cloud computing more complex. This paper proposes an NMDS (Network Manager based Dynamic Scheduling) for achieving a prominent resource allocation scheme for the users. The proposed system mainly focuses on dimensionality problems, where the conventional methods fail to address them. The proposed system introduced three –threshold mode of task based on its size STT, MTT, LTT (small, medium, large task thresholding). Along with it, task merging enables minimum energy consumption and response time. The proposed NMDS is compared with the existing Energy-efficient Dynamic Scheduling scheme (EDS) and Decentralized Virtual Machine Migration (DVM). With a Network Manager-based Dynamic Scheduling, the proposed model achieves excellence in resource allocation compared to the other existing models. The obtained results shows the proposed system effectively allocate the resources and achieves about 94% of energy efficient than the other models. The evaluation metrics taken for comparison are energy consumption, mean response time, percentage of resource utilization, and migration.Keywords
Cite This Article
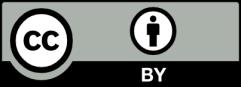