Open Access
ARTICLE
Optimal Artificial Intelligence Based Automated Skin Lesion Detection and Classification Model
1 Faculty of Engineering and the Built Environment, Department of Electrical and Electronics Engineering Technology, University of Johannesburg, Johannesburg, 2006, South Africa
2 Center for Artificial Intelligence and Research (CAIR), Chennai Institute of Technology, Chennai, 600069, India
3 Al-Nahrain Nanorenewable Energy Research Center, Al-Nahrain University, Baghdad, 64074, Iraq
* Corresponding Author: R. Surendran. Email:
Computer Systems Science and Engineering 2023, 44(1), 693-707. https://doi.org/10.32604/csse.2023.024154
Received 06 October 2021; Accepted 17 November 2021; Issue published 01 June 2022
Abstract
Skin lesions have become a critical illness worldwide, and the earlier identification of skin lesions using dermoscopic images can raise the survival rate. Classification of the skin lesion from those dermoscopic images will be a tedious task. The accuracy of the classification of skin lesions is improved by the use of deep learning models. Recently, convolutional neural networks (CNN) have been established in this domain, and their techniques are extremely established for feature extraction, leading to enhanced classification. With this motivation, this study focuses on the design of artificial intelligence (AI) based solutions, particularly deep learning (DL) algorithms, to distinguish malignant skin lesions from benign lesions in dermoscopic images. This study presents an automated skin lesion detection and classification technique utilizing optimized stacked sparse autoencoder (OSSAE) based feature extractor with backpropagation neural network (BPNN), named the OSSAE-BPNN technique. The proposed technique contains a multi-level thresholding based segmentation technique for detecting the affected lesion region. In addition, the OSSAE based feature extractor and BPNN based classifier are employed for skin lesion diagnosis. Moreover, the parameter tuning of the SSAE model is carried out by the use of sea gull optimization (SGO) algorithm. To showcase the enhanced outcomes of the OSSAE-BPNN model, a comprehensive experimental analysis is performed on the benchmark dataset. The experimental findings demonstrated that the OSSAE-BPNN approach outperformed other current strategies in terms of several assessment metrics.Keywords
Cite This Article
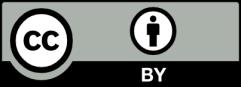
This work is licensed under a Creative Commons Attribution 4.0 International License , which permits unrestricted use, distribution, and reproduction in any medium, provided the original work is properly cited.