Open Access
ARTICLE
Lexicalized Dependency Paths Based Supervised Learning for Relation Extraction
New York University, New York, 10012, USA
* Corresponding Author: Huiyu Sun. Email:
Computer Systems Science and Engineering 2022, 43(3), 861-870. https://doi.org/10.32604/csse.2022.030759
Received 11 February 2022; Accepted 16 April 2022; Issue published 09 May 2022
Abstract
Log-linear models and more recently neural network models used for supervised relation extraction requires substantial amounts of training data and time, limiting the portability to new relations and domains. To this end, we propose a training representation based on the dependency paths between entities in a dependency tree which we call lexicalized dependency paths (LDPs). We show that this representation is fast, efficient and transparent. We further propose representations utilizing entity types and its subtypes to refine our model and alleviate the data sparsity problem. We apply lexicalized dependency paths to supervised learning using the ACE corpus and show that it can achieve similar performance level to other state-of-the-art methods and even surpass them on several categories.Keywords
Cite This Article
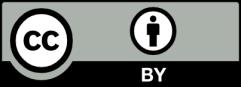