Open Access
ARTICLE
Image Inpainting Detection Based on High-Pass Filter Attention Network
1 Hunan Provincial Key Laboratory of Intelligent Processing of Big Data on Transportation, Changsha University of Science and Technology, Changsha, 410114, China
2 School of Computer and Communication Engineering, Changsha University of Science and Technology, Changsha, 410114, China
3 School of Computer Science and Engineering, Hunan University of Science and Technology, Xiangtan, 411004, China
4 Department of Computer Science, Texas Tech University, Lubbock, 79409, USA
* Corresponding Author: Feng Li. Email:
Computer Systems Science and Engineering 2022, 43(3), 1145-1154. https://doi.org/10.32604/csse.2022.027249
Received 13 January 2022; Accepted 11 March 2022; Issue published 09 May 2022
Abstract
Image inpainting based on deep learning has been greatly improved. The original purpose of image inpainting was to repair some broken photos, such as inpainting artifacts. However, it may also be used for malicious operations, such as destroying evidence. Therefore, detection and localization of image inpainting operations are essential. Recent research shows that high-pass filtering full convolutional network (HPFCN) is applied to image inpainting detection and achieves good results. However, those methods did not consider the spatial location and channel information of the feature map. To solve these shortcomings, we introduce the squeezed excitation blocks (SE) and propose a high-pass filter attention full convolutional network (HPACN). In feature extraction, we apply concurrent spatial and channel attention (scSE) to enhance feature extraction and obtain more information. Channel attention (cSE) is introduced in upsampling to enhance detection and localization. The experimental results show that the proposed method can achieve improvement on ImageNet.Keywords
Cite This Article
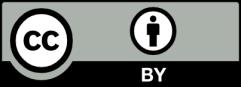
This work is licensed under a Creative Commons Attribution 4.0 International License , which permits unrestricted use, distribution, and reproduction in any medium, provided the original work is properly cited.