Open Access
ARTICLE
Deep Convolutional Neural Network Based Churn Prediction for Telecommunication Industry
1 Department of Computer Science, College of Computer, Qassim University, Buraydah, Saudi Arabia
2 Department of Computing, School of Electrical Engineering and Computer Science, National University of Sciences and Technology (NUST), Islamabad, Pakistan
* Corresponding Author: Ali Mustafa Qamar. Email:
Computer Systems Science and Engineering 2022, 43(3), 1255-1270. https://doi.org/10.32604/csse.2022.025029
Received 08 November 2021; Accepted 10 December 2021; Issue published 09 May 2022
Abstract
Currently, mobile communication is one of the widely used means of communication. Nevertheless, it is quite challenging for a telecommunication company to attract new customers. The recent concept of mobile number portability has also aggravated the problem of customer churn. Companies need to identify beforehand the customers, who could potentially churn out to the competitors. In the telecommunication industry, such identification could be done based on call detail records. This research presents an extensive experimental study based on various deep learning models, such as the 1D convolutional neural network (CNN) model along with the recurrent neural network (RNN) and deep neural network (DNN) for churn prediction. We use the mobile telephony churn prediction dataset obtained from customers-dna.com, containing the data for around 100,000 individuals, out of which 86,000 are non-churners, whereas 14,000 are churned customers. The imbalanced data are handled using undersampling and oversampling. The accuracy for CNN, RNN, and DNN is 91%, 93%, and 96%, respectively. Furthermore, DNN got 99% for ROC.Keywords
Cite This Article
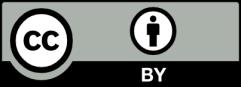
This work is licensed under a Creative Commons Attribution 4.0 International License , which permits unrestricted use, distribution, and reproduction in any medium, provided the original work is properly cited.