Open Access
ARTICLE
QL-CBR Hybrid Approach for Adapting Context-Aware Services
1 Ecole de Technologie Supérieure, Montréal, QCH3C1K3, Canada
2 Faculty of Exact and Applied Sciences, University of Oran 1, Oran, 31000, Algeria
3 Higher Institute of Sciences and Technologies, University of Gafsa, Gafsa, 2100, Tunisia
4 AL-Lith Computer College, Umm Al-Qura University, Al-Lith, 28434, Saudi Arabia
* Corresponding Author: Moeiz Miraoui. Email:
Computer Systems Science and Engineering 2022, 43(3), 1085-1098. https://doi.org/10.32604/csse.2022.024056
Received 01 October 2021; Accepted 11 November 2021; Issue published 09 May 2022
Abstract
A context-aware service in a smart environment aims to supply services according to user situational information, which changes dynamically. Most existing context-aware systems provide context-aware services based on supervised algorithms. Reinforcement algorithms are another type of machine-learning algorithm that have been shown to be useful in dynamic environments through trial-and-error interactions. They also have the ability to build excellent self-adaptive systems. In this study, we aim to incorporate reinforcement algorithms (Q-learning) into a context-aware system to provide relevant services based on a user’s dynamic context. To accelerate the convergence of reinforcement learning (RL) algorithms and provide the correct services in real situations, we propose a combination of the Q-learning and case-based reasoning (CBR) algorithms. We then analyze how the incorporation of CBR enables Q-learning to become more efficient and adapt to changing environments by continuously producing suitable services. Simulation results demonstrate the effectiveness of the proposed approach compared to the traditional CBR approach.Keywords
Cite This Article
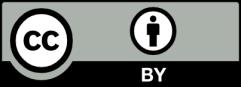
This work is licensed under a Creative Commons Attribution 4.0 International License , which permits unrestricted use, distribution, and reproduction in any medium, provided the original work is properly cited.