Open Access
ARTICLE
Multi-Site Air Pollutant Prediction Using Long Short Term Memory
Anna University, Chennai, 600066, Tamil Nadu, India
* Corresponding Author: Chitra Paulpandi. Email:
Computer Systems Science and Engineering 2022, 43(3), 1341-1355. https://doi.org/10.32604/csse.2022.023882
Received 25 September 2021; Accepted 08 November 2021; Issue published 09 May 2022
Abstract
The current pandemic highlights the significance and impact of air pollution on individuals. When it comes to climate sustainability, air pollution is a major challenge. Because of the distinctive nature, unpredictability, and great changeability in the reality of toxins and particulates, detecting air quality is a puzzling task. Simultaneously, the ability to predict or classify and monitor air quality is becoming increasingly important, particularly in urban areas, due to the well documented negative impact of air pollution on resident’s health and the environment. To better comprehend the current condition of air quality, this research proposes predicting air pollution levels from real-time data. This study proposes the use of deep learning techniques to forecast air pollution levels. Layers, activation functions, and a number of epochs were used to create the suggested Long Short-Term Memory (LSTM) network based neural layer design. The use of proposed Deep Learning as a structure for high-accuracy air quality prediction is investigated in this research and obtained better accuracy of nearly 82% compared to earlier records. Determining the Air Quality Index (AQI) and danger levels would assist the government in finding appropriate ways to authorize approaches to reduce pollutants and keep inhabitants informed about the findings.Keywords
Cite This Article
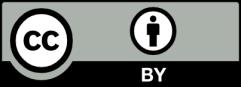
This work is licensed under a Creative Commons Attribution 4.0 International License , which permits unrestricted use, distribution, and reproduction in any medium, provided the original work is properly cited.