Open Access
ARTICLE
Spatio-Temporal Wind Speed Prediction Based on Variational Mode Decomposition
1 School of Computer and Science, Nanjing University of Information Science and Technology, Nanjing, 210044, China
2 Department of Electrical and Computer Engineering, University of Windsor, Windsor, N9B 3P4, Canada
* Corresponding Author: Yingnan Zhao. Email:
Computer Systems Science and Engineering 2022, 43(2), 719-735. https://doi.org/10.32604/csse.2022.027288
Received 13 January 2022; Accepted 21 March 2022; Issue published 20 April 2022
Abstract
Improving short-term wind speed prediction accuracy and stability remains a challenge for wind forecasting researchers. This paper proposes a new variational mode decomposition (VMD)-attention-based spatio-temporal network (VASTN) method that takes advantage of both temporal and spatial correlations of wind speed. First, VASTN is a hybrid wind speed prediction model that combines VMD, squeeze-and-excitation network (SENet), and attention mechanism (AM)-based bidirectional long short-term memory (BiLSTM). VASTN initially employs VMD to decompose the wind speed matrix into a series of intrinsic mode functions (IMF). Then, to extract the spatial features at the bottom of the model, each IMF employs an improved convolutional neural network algorithm based on channel AM, also known as SENet. Second, it combines BiLSTM and AM at the top layer to extract aggregated spatial features and capture temporal dependencies. Finally, VASTN accumulates the predictions of each IMF to obtain the predicted wind speed. This method employs VMD to reduce the randomness and instability of the original data before employing AM to improve prediction accuracy through mapping weight and parameter learning. Experimental results on real-world data demonstrate VASTN’s superiority over previous related algorithms.Keywords
Cite This Article
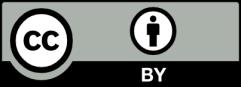