Open Access
ARTICLE
Pattern Recognition of Modulation Signal Classification Using Deep Neural Networks
1 Department of Electronics and Communication Engineering, KPR Institute of Engineering and Technology, Coimbatore, 641048, India
2 Department of Electronics and Communication Engineering, Saranathan College of Engineering, Trichy, 620012, India
3 Department of Computer Science and Engineering, Krishnasamy College of Engineering & Technology, Cuddalore, 607109, India
4 Department of Electronics and Communication Engineering, K. Ramakrishnan College of Engineering, Tiruchirappalli, 621112, India
5 Department of Computer Engineering, Sungkyul University, Anyang, Korea
6 Department of Applied Data Science, Noroff University College, Kristiansand, Norway
* Corresponding Author: Sangsoon Lim. Email:
Computer Systems Science and Engineering 2022, 43(2), 545-558. https://doi.org/10.32604/csse.2022.024239
Received 10 October 2021; Accepted 11 November 2021; Issue published 20 April 2022
Abstract
In recent times, pattern recognition of communication modulation signals has gained significant attention in several application areas such as military, civilian field, etc. It becomes essential to design a safe and robust feature extraction (FE) approach to efficiently identify the various signal modulation types in a complex platform. Several works have derived new techniques to extract the feature parameters namely instant features, fractal features, and so on. In addition, machine learning (ML) and deep learning (DL) approaches can be commonly employed for modulation signal classification. In this view, this paper designs pattern recognition of communication signal modulation using fractal features with deep neural networks (CSM-FFDNN). The goal of the CSM-FFDNN model is to classify the different types of digitally modulated signals. The proposed CSM-FFDNN model involves two major processes namely FE and classification. The proposed model uses Sevcik Fractal Dimension (SFD) technique to extract the fractal features from the digital modulated signals. Besides, the extracted features are fed into the DNN model for modulation signal classification. To improve the classification performance of the DNN model, a barnacles mating optimizer (BMO) is used for the hyperparameter tuning of the DNN model in such a way that the DNN performance can be raised. A wide range of simulations takes place to highlight the enhanced performance of the CSM-FFDNN model. The experimental outcomes pointed out the superior recognition rate of the CSM-FFDNN model over the recent state of art methods interms of different evaluation parameters.Keywords
Cite This Article
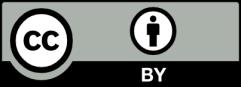
This work is licensed under a Creative Commons Attribution 4.0 International License , which permits unrestricted use, distribution, and reproduction in any medium, provided the original work is properly cited.