Open Access
ARTICLE
An Efficient Video Inpainting Approach Using Deep Belief Network
1 Department of Electronics and Communication Engineering, E.G.S. Pillay Engineering College, Nagapattinam, 611002, Tamilnadu, India
2 Department of Computer Science and Engineering, E.G.S. Pillay Engineering College, Nagapattinam, 611002, Tamilnadu, India
* Corresponding Author: M. Nuthal Srinivasan. Email:
Computer Systems Science and Engineering 2022, 43(2), 515-529. https://doi.org/10.32604/csse.2022.023109
Received 28 August 2021; Accepted 09 October 2021; Issue published 20 April 2022
Abstract
The video inpainting process helps in several video editing and restoration processes like unwanted object removal, scratch or damage rebuilding, and retargeting. It intends to fill spatio-temporal holes with reasonable content in the video. Inspite of the recent advancements of deep learning for image inpainting, it is challenging to outspread the techniques into the videos owing to the extra time dimensions. In this view, this paper presents an efficient video inpainting approach using beetle antenna search with deep belief network (VIA-BASDBN). The proposed VIA-BASDBN technique initially converts the videos into a set of frames and they are again split into a region of 5*5 blocks. In addition, the VIA-BASDBN technique involves the design of optimal DBN model, which receives input features from Local Binary Patterns (LBP) to categorize the blocks into smooth or structured regions. Furthermore, the weight vectors of the DBN model are optimally chosen by the use of BAS technique. Finally, the inpainting of the smooth and structured regions takes place using the mean and patch matching approaches respectively. The patch matching process depends upon the minimal Euclidean distance among the extracted SIFT features of the actual and references patches. In order to examine the effective outcome of the VIA-BASDBN technique, a series of simulations take place and the results denoted the promising performance.Keywords
Cite This Article
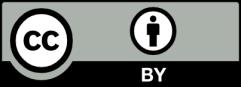