Open Access
ARTICLE
X-ray Image-Based COVID-19 Patient Detection Using Machine Learning-Based Techniques
1 Department of Information Technology, College of Computer, Qassim University, Buraidah, 52571, Saudi Arabia
2 Department of Electrical Engineering, College of Engineering & Information Technology, Onaizah Colleges, Onaizah, 51911, Saudi Arabia
3 Digital Image Processing Lab, Islamia College, Peshawar, 25120, KPK, Pakistan
4 Department of Computer Science, College of Computer and Information Sciences, Prince Sultan University, Riyadh, 11586, Saudi Arabia
5 Department of Electrical Engineering, National University of Computer and Emerging Sciences, Peshawar, Pakistan
* Corresponding Author: Muhammad Islam. Email:
Computer Systems Science and Engineering 2022, 43(2), 671-682. https://doi.org/10.32604/csse.2022.021812
Received 15 July 2021; Accepted 26 September 2021; Issue published 20 April 2022
Abstract
In early December 2019, the city of Wuhan, China, reported an outbreak of coronavirus disease (COVID-19), caused by a novel severe acute respiratory syndrome coronavirus-2 (SARS-CoV-2). On January 30, 2020, the World Health Organization (WHO) declared the outbreak a global pandemic crisis. In the face of the COVID-19 pandemic, the most important step has been the effective diagnosis and monitoring of infected patients. Identifying COVID-19 using Machine Learning (ML) technologies can help the health care unit through assistive diagnostic suggestions, which can reduce the health unit's burden to a certain extent. This paper investigates the possibilities of ML techniques in identifying/detecting COVID-19 patients including both conventional and exploring from chest X-ray images the effect of viral infection. This approach includes pre-processing, feature extraction, and classification. However, the features are extracted using the Histogram of Oriented (HOG) and Local Binary Pattern (LBP) feature descriptors. Furthermore, for the extracted features classification, six ML models of Support Vector Machine (SVM) and K-Nearest Neighbor (KNN) is used. Experimental results show that the diagnostic accuracy of random forest classifier (RFC) on extracted HOG plusLBP features is as high as 94% followed by SVM at 93%. The sensitivity of the K-nearest neighbour model has reached an accuracy of 88%. Overall, the predicted approach has shown higher classification accuracy and effective diagnostic performance. It is a highly useful tool for clinical practitioners and radiologists to help them in diagnosing and tracking the cases of COVID-19.Keywords
Cite This Article
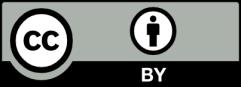
This work is licensed under a Creative Commons Attribution 4.0 International License , which permits unrestricted use, distribution, and reproduction in any medium, provided the original work is properly cited.