Open Access
ARTICLE
Support Vector Machine Based Handwritten Hindi Character Recognition and Summarization
1 Department of Computer Science & Engineering, Swami Keshvanand Institute of Technology, Management & Gramothan, Jaipur, Rajasthan, 302017, India
2 Department of Electrical Engineering, Sukkur IBA University, Pakistan
3 Department of Mechatronics Engineering, Jeju National University, Jeju, 63243, Korea
4 Department of Information Systems, College of Computer Science and Information Technology, King Faisal University, Saudi Arabia
5 Faculty of Computing & Information Technology, King Abdulaziz University, Rabigh, 21911, Saudi Arabia
* Corresponding Author: Sunil Dhankhar. Email:
Computer Systems Science and Engineering 2022, 43(1), 397-412. https://doi.org/10.32604/csse.2022.024059
Received 02 October 2021; Accepted 08 November 2021; Issue published 23 March 2022
Abstract
In today’s digital era, the text may be in form of images. This research aims to deal with the problem by recognizing such text and utilizing the support vector machine (SVM). A lot of work has been done on the English language for handwritten character recognition but very less work on the under-resourced Hindi language. A method is developed for identifying Hindi language characters that use morphology, edge detection, histograms of oriented gradients (HOG), and SVM classes for summary creation. SVM rank employs the summary to extract essential phrases based on paragraph position, phrase position, numerical data, inverted comma, sentence length, and keywords features. The primary goal of the SVM optimization function is to reduce the number of features by eliminating unnecessary and redundant features. The second goal is to maintain or improve the classification system’s performance. The experiment included news articles from various genres, such as Bollywood, politics, and sports. The proposed method’s accuracy for Hindi character recognition is 96.97%, which is good compared with baseline approaches, and system-generated summaries are compared to human summaries. The evaluated results show a precision of 72% at a compression ratio of 50% and a precision of 60% at a compression ratio of 25%, in comparison to state-of-the-art methods, this is a decent result.Keywords
Cite This Article
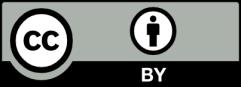