Open Access
ARTICLE
Conditional Probability Approach for Fault Detection in Photovoltaic Energy Farms
1 Electrical Engineering Department, Faculty of Engineering, Menoufia University, 32511, Egypt
2 Electrical Engineering Department, College of Engineering, Taif University, 21944, Saudi Arabia
* Corresponding Author: Nagy I. Elkalashy. Email:
(This article belongs to the Special Issue: Recent Trends in Software Engineering and Applications)
Computer Systems Science and Engineering 2022, 42(3), 1109-1120. https://doi.org/10.32604/csse.2022.023509
Received 11 September 2021; Accepted 12 October 2021; Issue published 08 February 2022
Abstract
Detection of electric faults in photovoltaic (PV) farms enhances a sustainable service continuity of farm energy generation. In this paper, a probabilistic function is introduced to detect the faults in the PV farms. The conditional probability functions are adopted to detect different fault conditions such as internal string faults, string-to-string faults, and string-to-negative terminal faults. As the diodes are important to make the PV farms in-service safely during the faults, the distribution currents of these faults are evaluated with different concepts of diode consideration as well as without considering any diode installation. This part of the study enhances the diode utilization in the PV farms concerning the protection point of view. The PV string currents are used as inputs to the conditional probability detection algorithms. However, the setting of the fault detection technique is not portable for the other PV systems due to broad ranges of PV system ratings. To accordingly generalize the proposed fault detection algorithm, the PV string currents are first normalized to the total array current for universally applying the detection function at different PV string ratings. The limiting fault resistances are evaluated to show the sensitivity of the proposed fault detector. The results ensure the application of the proposed probabilistic detection function for PV farms.Keywords
Cite This Article
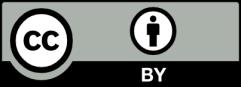
This work is licensed under a Creative Commons Attribution 4.0 International License , which permits unrestricted use, distribution, and reproduction in any medium, provided the original work is properly cited.