Open Access
ARTICLE
CNN and Fuzzy Rules Based Text Detection and Recognition from Natural Scenes
1 Department of Computer Science and Engineering, University College Engineering, BIT Campus, Anna University, Trichy, 620021, India
2 Department of Computer Science and Engineering, University College of Engineering, Ariyalur, 621704, India
3 Department of Computer Science and Engineering, University College of Engineering, Panruti, 607106, India
* Corresponding Author: T. Mithila. Email:
Computer Systems Science and Engineering 2022, 42(3), 1165-1179. https://doi.org/10.32604/csse.2022.023308
Received 02 September 2021; Accepted 09 October 2021; Issue published 08 February 2022
Abstract
In today’s real world, an important research part in image processing is scene text detection and recognition. Scene text can be in different languages, fonts, sizes, colours, orientations and structures. Moreover, the aspect ratios and layouts of a scene text may differ significantly. All these variations appear assignificant challenges for the detection and recognition algorithms that are considered for the text in natural scenes. In this paper, a new intelligent text detection and recognition method for detectingthe text from natural scenes and forrecognizing the text by applying the newly proposed Conditional Random Field-based fuzzy rules incorporated Convolutional Neural Network (CR-CNN) has been proposed. Moreover, we have recommended a new text detection method for detecting the exact text from the input natural scene images. For enhancing the presentation of the edge detection process, image pre-processing activities such as edge detection and color modeling have beenapplied in this work. In addition, we have generated new fuzzy rules for making effective decisions on the processes of text detection and recognition. The experiments have been directedusing the standard benchmark datasets such as the ICDAR 2003, the ICDAR 2011, the ICDAR 2005 and the SVT and have achieved better detection accuracy intext detection and recognition. By using these three datasets, five different experiments have been conducted for evaluating the proposed model. And also, we have compared the proposed system with the other classifiers such as the SVM, the MLP and the CNN. In these comparisons, the proposed model has achieved better classification accuracywhen compared with the other existing works.Keywords
Cite This Article
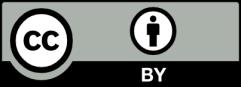