Open Access
ARTICLE
Planetscope Nanosatellites Image Classification Using Machine Learning
Department of Computer Science, College of Computer and Information Sciences, Majmaah University, ALMajmaah, 11952, Saudi Arabia
* Corresponding Author: Mohd Anul Haq. Email:
(This article belongs to the Special Issue: Innovation in Image Processing with Programmable Logic gates)
Computer Systems Science and Engineering 2022, 42(3), 1031-1046. https://doi.org/10.32604/csse.2022.023221
Received 31 August 2021; Accepted 09 October 2021; Issue published 08 February 2022
Abstract
To adopt sustainable crop practices in changing climate, understanding the climatic parameters and water requirements with vegetation is crucial on a spatiotemporal scale. The Planetscope (PS) constellation of more than 130 nanosatellites from Planet Labs revolutionize the high-resolution vegetation assessment. PS-derived Normalized Difference Vegetation Index (NDVI) maps are one of the highest resolution data that can transform agricultural practices and management on a large scale. High-resolution PS nanosatellite data was utilized in the current study to monitor agriculture’s spatiotemporal assessment for the Al-Qassim region, Kingdom of Saudi Arabia (KSA). The time series of NDVI was utilized to assess the vegetation pattern change in the study area. The current study area has sparse vegetation, and exposed soil exhibits brightness due to low soil moisture, constraining NDVI. Therefore, a machine learning (ML) based Random Forest (RF) classification model was used to compare the vegetation extent and computational cost of NDVI. The RF model has been compared with NDVI in the current investigation. It is one of the most precise classification methods because it can model the complexity of input variables, handle outliers, treat noise effectively, and avoid overfitting. Multinomial Logistic Regression (MLR) was implemented to compare the performance of both NDVI and RF-based classification. RF model provided good accuracy (98%) for all vegetation classes based on user accuracy, producer accuracy, and kappa coefficient.Keywords
Cite This Article
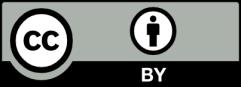