Open Access
ARTICLE
Rice Leaves Disease Diagnose Empowered with Transfer Learning
1 College of Computer and Information Sciences, Jouf University, Sakaka, 72341, Saudi Arabia
2 Department of Computer Science, Faculty of Computers and Artificial Intelligence, Cairo University, 12613, Egypt
3 School of Computer Science, National College of Business Administration and Economics, Lahore, 54000, Pakistan
4 Department of Computer Science, Lahore Garrison University, Lahore, 54000, Pakistan
5 Pattern Recognition and Machine Learning Lab, Department of Software, Gachon University, Seongnam, 13557, Korea
6 Department of Information System, Faculty of Computing and Information Technology, King Abdulaziz University, Jeddah, 21589, Saudi Arabia
* Corresponding Author: Muhammad Adnan Khan. Email:
Computer Systems Science and Engineering 2022, 42(3), 1001-1014. https://doi.org/10.32604/csse.2022.022017
Received 23 July 2021; Accepted 24 August 2021; Issue published 08 February 2022
Abstract
In the agricultural industry, rice infections have resulted in significant productivity and economic losses. The infections must be recognized early on to regulate and mitigate the effects of the attacks. Early diagnosis of disease severity effects or incidence can preserve production from quantitative and qualitative losses, reduce pesticide use, and boost ta country’s economy. Assessing the health of a rice plant through its leaves is usually done as a manual ocular exercise. In this manuscript, three rice plant diseases: Bacterial leaf blight, Brown spot, and Leaf smut, were identified using the Alexnet Model. Our research shows that any reduction in rice plants will have a significant beneficial impact on alleviating global food hunger by increasing supply, lowering prices, and reducing production's environmental impact that affects the economy of any country. Farmers would be able to get more exact and faster results with this technology, allowing them to administer the most acceptable treatment available. By Using Alex Net, the proposed approach achieved a 99.0% accuracy rate for diagnosing rice leaves disease.Keywords
Cite This Article
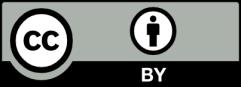