Open Access
ARTICLE
Ensemble Nonlinear Support Vector Machine Approach for Predicting Chronic Kidney Diseases
1 Department of Computer Science and Engineering, Sri Shakthi Institute of Engineering and Technology, Coimbatore, 641062, India
2 Department of Computer Science and Engineering, Kongu Engineering College, Perundurai, 638060, India
3 School of Computing Science and Engineering, VIT Bhopal University, Bhopal, 466114, India
4 Department of Computer Science and Engineering, Sri Krishna College of Engineering and Technology, Coimbatore, 641008, India
5 Department of ECE, Sri Krishna College of Engineering and Technology, Coimbatore, 641008, India
6 Department of Applied Cybernetics, Faculty of Science, University of Hradec Králové, 50003, Hradec Králové, Czech Republic
* Corresponding Author: S. Prakash. Email:
Computer Systems Science and Engineering 2022, 42(3), 1273-1287. https://doi.org/10.32604/csse.2022.021784
Received 14 July 2021; Accepted 22 September 2021; Issue published 08 February 2022
Abstract
Urban living in large modern cities exerts considerable adverse effects on health and thus increases the risk of contracting several chronic kidney diseases (CKD). The prediction of CKDs has become a major task in urbanized countries. The primary objective of this work is to introduce and develop predictive analytics for predicting CKDs. However, prediction of huge samples is becoming increasingly difficult. Meanwhile, MapReduce provides a feasible framework for programming predictive algorithms with map and reduce functions. The relatively simple programming interface helps solve problems in the scalability and efficiency of predictive learning algorithms. In the proposed work, the iterative weighted map reduce framework is introduced for the effective management of large dataset samples. A binary classification problem is formulated using ensemble nonlinear support vector machines and random forests. Thus, instead of using the normal linear combination of kernel activations, the proposed work creates nonlinear combinations of kernel activations in prototype examples. Furthermore, different descriptors are combined in an ensemble of deep support vector machines, where the product rule is used to combine probability estimates of different classifiers. Performance is evaluated in terms of the prediction accuracy and interpretability of the model and the results.Keywords
Cite This Article
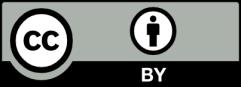
This work is licensed under a Creative Commons Attribution 4.0 International License , which permits unrestricted use, distribution, and reproduction in any medium, provided the original work is properly cited.