Open Access
ARTICLE
Fusion Recommendation System Based on Collaborative Filtering and Knowledge Graph
1 School of Artificial Intelligence, Wuxi Vocational College of Science and Technology, Wuxi, 214028, China
2 School of Computer Science and Technology, Harbin Institute of Technology, Weihai, 264209, China
3 School of Astronautics, Harbin Institute of Technology, Harbin, 150001, China
4 Department of Mathematics, Harbin Institute of Technology, Weihai, 264209, China
5 School of Mathematical Sciences, University College Dublin, Dublin, Dublin4, Ireland
* Corresponding Author: Dongjie Zhu. Email:
Computer Systems Science and Engineering 2022, 42(3), 1133-1146. https://doi.org/10.32604/csse.2022.021525
Received 05 July 2021; Accepted 14 September 2021; Issue published 08 February 2022
Abstract
The recommendation algorithm based on collaborative filtering is currently the most successful recommendation method. It recommends items to the user based on the known historical interaction data of the target user. Furthermore, the combination of the recommended algorithm based on collaborative filtration and other auxiliary knowledge base is an effective way to improve the performance of the recommended system, of which the Co-Factorization Model (CoFM) is one representative research. CoFM, a fusion recommendation model combining the collaborative filtering model FM and the graph embedding model TransE, introduces the information of many entities and their relations in the knowledge graph into the recommendation system as effective auxiliary information. It can effectively improve the accuracy of recommendations and alleviate the problem of sparse user historical interaction data. Unfortunately, the graph-embedded model TransE used in the CoFM model cannot solve the 1-N, N-1, and N-N problems well. To tackle this problem, a novel fusion recommendation model Joint Factorization Machines and TransH Model (JFMH) is proposed, which improves CoFM by replacing the TransE model with TransH model. A large number of experiments on two widely used benchmark data sets show that compared with CoFM, JFMH has improved performance in terms of item recommendation and knowledge graph completion, and is more competitive than multiple baseline methods.Keywords
Cite This Article
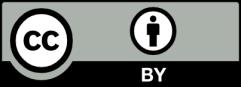