Open Access
ARTICLE
Modified Visual Geometric Group Architecture for MRI Brain Image Classification
Department of Electronics and Communication Engineering, SRM Institute of Science and Technology, Kattankulathur, Chennai, 603203, India
* Corresponding Author: N. Veni. Email:
Computer Systems Science and Engineering 2022, 42(2), 825-835. https://doi.org/10.32604/csse.2022.022318
Received 03 August 2021; Accepted 15 September 2021; Issue published 04 January 2022
Abstract
The advancement of automated medical diagnosis in biomedical engineering has become an important area of research. Image classification is one of the diagnostic approaches that do not require segmentation which can draw quicker inferences. The proposed non-invasive diagnostic support system in this study is considered as an image classification system where the given brain image is classified as normal or abnormal. The ability of deep learning allows a single model for feature extraction as well as classification whereas the rational models require separate models. One of the best models for image localization and classification is the Visual Geometric Group (VGG) model. In this study, an efficient modified VGG architecture for brain image classification is developed using transfer learning. The pooling layer is modified to enhance the classification capability of VGG architecture. Results show that the modified VGG architecture outperforms the conventional VGG architecture with a 5% improvement in classification accuracy using 16 layers on MRI images of the REpository of Molecular BRAin Neoplasia DaTa (REMBRANDT) database.Keywords
Cite This Article
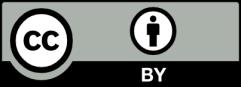
This work is licensed under a Creative Commons Attribution 4.0 International License , which permits unrestricted use, distribution, and reproduction in any medium, provided the original work is properly cited.