Open Access
ARTICLE
Industrial Centric Node Localization and Pollution Prediction Using Hybrid Swarm Techniques
1 Department of Electronics and Communication Engineering, Anna University, University College of Engineering Dindigul, Dindigul, 624622, India
2 Department of Computer Science and Engineering, Anna University, University College of Engineering Dindigul, Dindigul, 624622, India
3 Department of Computer Science and Engineering, Kongu Engineering College, Perundurai, 638060, India
4 School of Computing Science and Engineering, VIT Bhopal University, Bhopal, 466114, India
5 Department of Computer Science and Engineering, Sri Krishna College of Engineering and Technology, Coimbatore, 641008, India
6 Department of Information Technology, Sri Krishna College of Engineering and Technology, Coimbatore, 641008, India
* Corresponding Author: R. Saravana Ram. Email:
Computer Systems Science and Engineering 2022, 42(2), 545-460. https://doi.org/10.32604/csse.2022.021681
Received 10 July 2021; Accepted 30 August 2021; Issue published 04 January 2022
Abstract
Major fields such as military applications, medical fields, weather forecasting, and environmental applications use wireless sensor networks for major computing processes. Sensors play a vital role in emerging technologies of the 20th century. Localization of sensors in needed locations is a very serious problem. The environment is home to every living being in the world. The growth of industries after the industrial revolution increased pollution across the environment. Owing to recent uncontrolled growth and development, sensors to measure pollution levels across industries and surroundings are needed. An interesting and challenging task is choosing the place to fit the sensors. Many meta-heuristic techniques have been introduced in node localization. Swarm intelligent algorithms have proven their efficiency in many studies on localization problems. In this article, we introduce an industrial-centric approach to solve the problem of node localization in the sensor network. First, our work aims at selecting industrial areas in the sensed location. We use random forest regression methodology to select the polluted area. Then, the elephant herding algorithm is used in sensor node localization. These two algorithms are combined to produce the best standard result in localizing the sensor nodes. To check the proposed performance, experiments are conducted with data from the KDD Cup 2018, which contain the name of 35 stations with concentrations of air pollutants such as PM, SO2, CO, NO2, and O3. These data are normalized and tested with algorithms. The results are comparatively analyzed with other swarm intelligence algorithms such as the elephant herding algorithm, particle swarm optimization, and machine learning algorithms such as decision tree regression and multi-layer perceptron. Results can indicate our proposed algorithm can suggest more meaningful locations for localizing the sensors in the topology. Our proposed method achieves a lower root mean square value with 0.06 to 0.08 for localizing with Stations 1 to 5.Keywords
Cite This Article
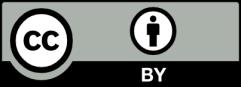