Open Access
ARTICLE
A Prediction Method of Fracture Toughness of Nickel-Based Superalloys
1 School of Computer, Beijing Information Science and Technology University, Beijing, 100101, China
2 Department of Information Science, University of Arkansas at Little Rock, Arkansas, 72204, USA
* Corresponding Author: Yabin Xu. Email:
Computer Systems Science and Engineering 2022, 42(1), 121-132. https://doi.org/10.32604/csse.2022.022758
Received 18 August 2021; Accepted 24 September 2021; Issue published 02 December 2021
Abstract
Fracture toughness plays a vital role in damage tolerance design of materials and assessment of structural integrity. To solve these problems of complexity, time-consuming, and low accuracy in obtaining the fracture toughness value of nickel-based superalloys through experiments. A combination prediction model is proposed based on the principle of materials genome engineering, the fracture toughness values of nickel-based superalloys at different temperatures, and different compositions can be predicted based on the existing experimental data. First, to solve the problem of insufficient feature extraction based on manual experience, the Deep Belief Network (DBN) is used to extract features, and an attention mechanism module is introduced. To achieve the purpose of strengthening the important features, an attention weight is assigned to each feature according to the importance of the feature. Then, the feature vectors obtained by the DBN module based on the Attention mechanism (A-DBN) are spliced with the original features. Thus, the prediction accuracy of the model is improved by extracting high-order combined features and low-order linear features between input and output data. Finally, the spliced feature vectors are put into the Support Vector Regression (SVR) model to further improve the regression prediction ability of the model. The results of the contrast experiment show that the model can effectively improve the prediction accuracy of the fracture toughness value of nickel-based superalloys.Keywords
Cite This Article
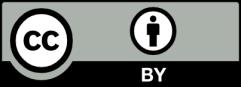