Open Access
ARTICLE
Make U-Net Greater: An Easy-to-Embed Approach to Improve Segmentation Performance Using Hypergraph
1 Chengdu Institute of Computer Application, University of Chinese Academy of Sciences, Chengdu, 610041, China
2 School of Computer Science, Chengdu University of Information Technology, Chengdu, 610225, China
3 University of Chinese Academy of Sciences, Beijing, 100049, China
4 Department of Experimental Rheumatology, Nijmegen, 6525GA, Netherlands
* Corresponding Author: Zhe Cui. Email:
Computer Systems Science and Engineering 2022, 42(1), 319-333. https://doi.org/10.32604/csse.2022.022314
Received 02 August 2021; Accepted 03 September 2021; Issue published 02 December 2021
Abstract
Cardiac anatomy segmentation is essential for cardiomyopathy clinical diagnosis and treatment planning. Thus, accurate delineation of target volumes at risk in cardiac anatomy is important. However, manual delineation is a time-consuming and labor-intensive process for cardiologists and has been shown to lead to significant inter-and intra-practitioner variability. Thus, computer-aided or fully automatic segmentation methods are required. They can significantly economize on manpower and improve treatment efficiency. Recently, deep convolutional neural network (CNN) based methods have achieved remarkable successes in various kinds of vision tasks, such as classification, segmentation and object detection. Semantic segmentation can be considered as a pixel-wise task, it requires high-level abstract semantics information while maintaining spatial detail contexts. Long-range context information plays a crucial role in this scenario. However, the traditional convolution kernel only provides the local and small size of the receptive field. To address the problem, we propose a plug-and-play module aggregating both local and global information (aka LGIA module) to capture the high-order relationship between nodes that are far apart. We incorporate both local and global correlations into hypergraph which is able to capture high-order relationships between nodes via the concept of a hyperedge connecting a subset of nodes. The local correlation considers neighborhood nodes that are spatially adjacent and similar in the same CNN feature maps of magnetic resonance (MR) image; and the global correlation is searched from a batch of CNN feature maps of MR images in feature space. The influence of these two correlations on semantic segmentation is complementary. We validated our LGIA module on various CNN segmentation models with the cardiac MR images dataset. Experimental results demonstrate that our approach outperformed several baseline models.Keywords
Cite This Article
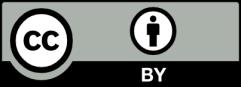
This work is licensed under a Creative Commons Attribution 4.0 International License , which permits unrestricted use, distribution, and reproduction in any medium, provided the original work is properly cited.