Open Access
ARTICLE
RDA- CNN: Enhanced Super Resolution Method for Rice Plant Disease Classification
1 Department of Computer Science and Engineering, Coimbatore Institute of Technology, Coimbatore, 641014, India
2 Department of Information Technology, Coimbatore Institute of Technology, Coimbatore, 641014, India
* Corresponding Author: K. Sathya. Email:
Computer Systems Science and Engineering 2022, 42(1), 33-47. https://doi.org/10.32604/csse.2022.022206
Received 29 July 2021; Accepted 30 August 2021; Issue published 02 December 2021
Abstract
In the field of agriculture, the development of an early warning diagnostic system is essential for timely detection and accurate diagnosis of diseases in rice plants. This research focuses on identifying the plant diseases and detecting them promptly through the advancements in the field of computer vision. The images obtained from in-field farms are typically with less visual information. However, there is a significant impact on the classification accuracy in the disease diagnosis due to the lack of high-resolution crop images. We propose a novel Reconstructed Disease Aware–Convolutional Neural Network (RDA-CNN), inspired by recent CNN architectures, that integrates image super resolution and classification into a single model for rice plant disease classification. This network takes low-resolution images of rice crops as input and employs the super resolution layers to transform low-resolution images to super-resolution images to recover appearance such as spots, rot, and lesion on different parts of the rice plants. Extensive experimental results indicated that the proposed RDA-CNN method performs well under diverse aspects generating visually pleasing images and outperforms better than other conventional Super Resolution (SR) methods. Furthermore, these super-resolution images are subsequently passed through deep classification layers for disease classification. The results demonstrate that the RDA- CNN significantly boosts the classification performance by nearly 4–6% compared with the baseline architectures.Keywords
Cite This Article
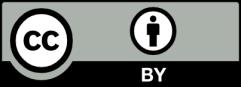
This work is licensed under a Creative Commons Attribution 4.0 International License , which permits unrestricted use, distribution, and reproduction in any medium, provided the original work is properly cited.