Open Access
ARTICLE
Towards Improving Predictive Statistical Learning Model Accuracy by Enhancing Learning Technique
1 Statistics Department, Faculty of Science, King Abdulaziz University, Jeddah, 21589, Saudi Arabia
2 Information Technology Department, Faculty of Computing and Information Technology, King Abdulaziz University, Jeddah, 21589, Saudi Arabia
3 Mathematics Department, Faculty of Science, Al-Azhar University, Naser City, 11884, Egypt
4 Centre of Artificial Intelligence for Precision Medicines, King Abdulaziz University, Jeddah, 21589, Saudi Arabia
5 Educational Technology Department, Educational Graduate Studies Faculty, King Abdulaziz University, Jeddah, 21589, Saudi Arabia
6 Computer Science Department, Faculty of Computers and Information, South Valley University, Qena, 83523, Egypt
* Corresponding Author: Mahmoud Ragab. Email:
Computer Systems Science and Engineering 2022, 42(1), 303-318. https://doi.org/10.32604/csse.2022.022152
Received 29 July 2021; Accepted 30 August 2021; Issue published 02 December 2021
Abstract
The accuracy of the statistical learning model depends on the learning technique used which in turn depends on the dataset’s values. In most research studies, the existence of missing values (MVs) is a vital problem. In addition, any dataset with MVs cannot be used for further analysis or with any data driven tool especially when the percentage of MVs are high. In this paper, the authors propose a novel algorithm for dealing with MVs depending on the feature selection (FS) of similarity classifier with fuzzy entropy measure. The proposed algorithm imputes MVs in cumulative order. The candidate feature to be manipulated is selected using similarity classifier with Parkash’s fuzzy entropy measure. The predictive model to predict MVs within the candidate feature is the Bayesian Ridge Regression (BRR) technique. Furthermore, any imputed features will be incorporated within the BRR equation to impute the MVs in the next chosen incomplete feature. The proposed algorithm was compared against some practical state-of-the-art imputation methods by conducting an experiment on four medical datasets which were gathered from several databases repository with MVs generated from the three missingness mechanisms. The evaluation metrics of mean absolute error (MAE), root mean square error (RMSE) and coefficient of determination (R2 score) were used to measure the performance. The results exhibited that performance vary depending on the size of the dataset, amount of MVs and the missingness mechanism type. Moreover, compared to other methods, the results showed that the proposed method gives better accuracy and less error in most cases.Keywords
Cite This Article
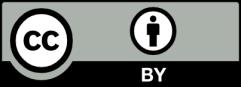