Open Access
ARTICLE
Autonomous Unbiased Study Group Formation Algorithm for Rapid Knowledge Propagation
1 Department of Computer Science, Babcock University, Ogun, Nigeria
2 Department of Computer Science, Admiralty University, Delta, Nigeria
3 Department of Computer Science, National Open University, Nigeria
4 Department of Computer Science and Informatics, Alex-Ekwueme Federal University, Ebonyi, Nigeria
5 Department of Cyber Security, Admiralty University, Delta, Nigeria
6 Department of Computer Science, Caleb University, Imota, Lagos, Nigeria
7 Department of Computer Science and Information Technology, Petroleum Training Institute, Delta, Nigeria
8 Department of Computer Science, Tai Solarin University of Education, Ogun, Nigeria
* Corresponding Author: Monday Eze. Email:
(This article belongs to the Special Issue: Advances in Computational Intelligence and its Applications)
Computer Systems Science and Engineering 2022, 42(1), 15-31. https://doi.org/10.32604/csse.2022.021964
Received 22 July 2021; Accepted 23 August 2021; Issue published 02 December 2021
Abstract
Knowledge propagation is a necessity, both in academics and in the industry. The focus of this work is on how to achieve rapid knowledge propagation using collaborative study groups. The practice of knowledge sharing in study groups finds relevance in conferences, workshops, and class rooms. Unfortunately, there appears to be only few researches on empirical best practices and techniques on study groups formation, especially for achieving rapid knowledge propagation. This work bridges this gap by presenting a workflow driven computational algorithm for autonomous and unbiased formation of study groups. The system workflow consists of a chronology of stages, each made of distinct steps. Two of the most important steps, subsumed within the algorithmic stage, are the algorithms that resolve the decisional problem of number of study groups to be formed, as well as the most effective permutation of the study group participants to form collaborative pairs. This work contributes a number of new algorithmic concepts, such as autonomous and unbiased matching, exhaustive multiplication technique, twisted round-robin transversal, equilibrium summation, among others. The concept of autonomous and unbiased matching is centered on the constitution of study groups and pairs purely based on the participants’ performances in an examination, rather than through any external process. As part of practical demonstration of this work, study group formation as well as unbiased pairing were fully demonstrated for a collaborative learning size of forty (40) participants, and partially for study groups of 50, 60 and 80 participants. The quantitative proof of this work was done through the technique called equilibrium summation, as well as the calculation of inter-study group Pearson Correlation Coefficients, which resulted in values higher than 0.9 in all cases. Real life experimentation was carried out while teaching Object-Oriented Programming to forty (40) undergraduates between February and May 2021. Empirical result showed that the performance of the learners was improved appreciably. This work will therefore be of immense benefit to the industry, academics and research community involved in collaborative learning.Keywords
Cite This Article
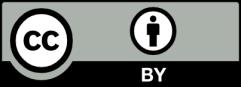
This work is licensed under a Creative Commons Attribution 4.0 International License , which permits unrestricted use, distribution, and reproduction in any medium, provided the original work is properly cited.