Open Access
ARTICLE
Aero-Engine Surge Fault Diagnosis Using Deep Neural Network
1 School of Computer Science, Southwest Petroleum University, Chengdu, 610500, China
2 Sichuan Normal University, Chengdu, 610066, China
3 AECC Sichuan Gas Turbine Establishment, MianYang, 621700, China
4 Brunel University London, Uxbridge, Middlesex, UB83PH, United Kingdom
* Corresponding Author: Bin Lin. Email:
Computer Systems Science and Engineering 2022, 42(1), 351-360. https://doi.org/10.32604/csse.2022.021132
Received 28 June 2021; Accepted 29 July 2021; Issue published 02 December 2021
Abstract
Deep learning techniques have outstanding performance in feature extraction and model fitting. In the field of aero-engine fault diagnosis, the introduction of deep learning technology is of great significance. The aero-engine is the heart of the aircraft, and its stable operation is the primary guarantee of the aircraft. In order to ensure the normal operation of the aircraft, it is necessary to study and diagnose the faults of the aero-engine. Among the many engine failures, the one that occurs more frequently and is more hazardous is the wheeze, which often poses a great threat to flight safety. On the basis of analyzing the mechanism of aero-engine surge, an aero-engine surge fault diagnosis method based on deep learning technology is proposed. In this paper, key sensor data are obtained by analyzing different engine sensor data. An aero-engine surge dataset acquisition algorithm (ASDA) is proposed to sample the fault and normal points to generate the training set, validation set and test set. Based on neural network models such as one-dimensional convolutional neural network (1D-CNN), convolutional neural network (RNN), and long-short memory neural network (LSTM), different neural network optimization algorithms are selected to achieve fault diagnosis and classification. The experimental results show that the deep learning technique has good effect in aero-engine surge fault diagnosis. The aero-engine surge fault diagnosis network (ASFDN) proposed in this paper achieves better results. Through training, the network achieves more than 99% classification accuracy for the test set.Keywords
Cite This Article
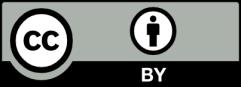
This work is licensed under a Creative Commons Attribution 4.0 International License , which permits unrestricted use, distribution, and reproduction in any medium, provided the original work is properly cited.