Open Access
ARTICLE
Defect Prediction Using Akaike and Bayesian Information Criterion
1 Department of Information Technology, College of Computer, Qassim University, Buraydah, Saudi Arabia
2 Department of Electrical and Computer Engineering, Air University, Islamabad, Pakistan
* Corresponding Author: Saleh Albahli. Email:
(This article belongs to the Special Issue: Recent Trends in Software Engineering and Applications)
Computer Systems Science and Engineering 2022, 41(3), 1117-1127. https://doi.org/10.32604/csse.2022.021750
Received 13 July 2021; Accepted 18 August 2021; Issue published 10 November 2021
Abstract
Data available in software engineering for many applications contains variability and it is not possible to say which variable helps in the process of the prediction. Most of the work present in software defect prediction is focused on the selection of best prediction techniques. For this purpose, deep learning and ensemble models have shown promising results. In contrast, there are very few researches that deals with cleaning the training data and selection of best parameter values from the data. Sometimes data available for training the models have high variability and this variability may cause a decrease in model accuracy. To deal with this problem we used the Akaike information criterion (AIC) and the Bayesian information criterion (BIC) for selection of the best variables to train the model. A simple ANN model with one input, one output and two hidden layers was used for the training instead of a very deep and complex model. AIC and BIC values are calculated and combination for minimum AIC and BIC values to be selected for the best model. At first, variables were narrowed down to a smaller number using correlation values. Then subsets for all the possible variable combinations were formed. In the end, an artificial neural network (ANN) model was trained for each subset and the best model was selected on the basis of the smallest AIC and BIC value. It was found that combination of only two variables’ ns and entropy are best for software defect prediction as it gives minimum AIC and BIC values. While, nm and npt is the worst combination and gives maximum AIC and BIC values.Keywords
Cite This Article
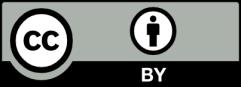
This work is licensed under a Creative Commons Attribution 4.0 International License , which permits unrestricted use, distribution, and reproduction in any medium, provided the original work is properly cited.