Open Access
ARTICLE
Emotion Recognition with Capsule Neural Network
1 School of Information and Communication Technology, Hanoi University of Science and Technology, Hanoi, 10000, Vietnam
2 Faculty of Information Technology, University of Transport and Communications, Hanoi, 10000, Vietnam
* Corresponding Author: Quang H. Nguyen. Email:
Computer Systems Science and Engineering 2022, 41(3), 1083-1098. https://doi.org/10.32604/csse.2022.021635
Received 08 July 2021; Accepted 13 August 2021; Issue published 10 November 2021
Abstract
For human-machine communication to be as effective as human-to-human communication, research on speech emotion recognition is essential. Among the models and the classifiers used to recognize emotions, neural networks appear to be promising due to the network’s ability to learn and the diversity in configuration. Following the convolutional neural network, a capsule neural network (CapsNet) with inputs and outputs that are not scalar quantities but vectors allows the network to determine the part-whole relationships that are specific 6 for an object. This paper performs speech emotion recognition based on CapsNet. The corpora for speech emotion recognition have been augmented by adding white noise and changing voices. The feature parameters of the recognition system input are mel spectrum images along with the characteristics of the sound source, vocal tract and prosody. For the German emotional corpus EMO-DB, the average accuracy score for 4 emotions, neutral, boredom, anger and happiness, is 99.69%. For Vietnamese emotional corpus BKEmo, this score is 94.23% for 4 emotions, neutral, sadness, anger and happiness. The accuracy score is highest when combining all the above feature parameters, and this score increases significantly when combining mel spectrum images with the features directly related to the fundamental frequency.Keywords
Cite This Article
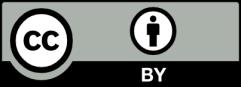
This work is licensed under a Creative Commons Attribution 4.0 International License , which permits unrestricted use, distribution, and reproduction in any medium, provided the original work is properly cited.