Open Access
ARTICLE
Prediction of Covid-19 Based on Chest X-Ray Images Using Deep Learning with CNN
1 Department of Electrical and Computer Engineering, North South University, Bashundhara, Dhaka, 1229, Bangladesh
2 Department of Computer Science, College of Computers and Information Technology, Taif University, P. O. Box 11099, Taif 21944, Saudi Arabia
* Corresponding Author: Mohammad Monirujjaman Khan. Email:
Computer Systems Science and Engineering 2022, 41(3), 1223-1240. https://doi.org/10.32604/csse.2022.021563
Received 07 July 2021; Accepted 17 August 2021; Issue published 10 November 2021
Abstract
The COVID-19 pandemic has caused trouble in people’s daily lives and ruined several economies around the world, killing millions of people thus far. It is essential to screen the affected patients in a timely and cost-effective manner in order to fight this disease. This paper presents the prediction of COVID-19 with Chest X-Ray images, and the implementation of an image processing system operated using deep learning and neural networks. In this paper, a Deep Learning, Machine Learning, and Convolutional Neural Network-based approach for predicting Covid-19 positive and normal patients using Chest X-Ray pictures is proposed. In this study, machine learning tools such as TensorFlow were used for building and training neural nets. Scikit-learn was used for machine learning from end to end. Various deep learning features are used, such as Conv2D, Dense Net, Dropout, Maxpooling2D for creating the model. The proposed approach had a classification accuracy of 96.43 percent and a validation accuracy of 98.33 percent after training and testing the X-Ray pictures. Finally, a web application has been developed for general users, which will detect chest x-ray images either as covid or normal. A GUI application for the Covid prediction framework was run. A chest X-ray image can be browsed and fed into the program by medical personnel or the general public.Keywords
Cite This Article
Citations
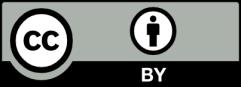
This work is licensed under a Creative Commons Attribution 4.0 International License , which permits unrestricted use, distribution, and reproduction in any medium, provided the original work is properly cited.