Open Access
ARTICLE
Smart COVID-3D-SCNN: A Novel Method to Classify X-ray Images of COVID-19
1 College of Technological Innovation, Zayed University, AbuDhabi Campus, UAE
2 School of Artificial Intelligence, Xidian University, Xian, 710071, China
3 Computer Science Department, Community College, King Saud University, P.O. Box 28095, Riyadh 11437, Saudi Arabia
4 Nathan campus, Griffith University, Brisbane, Australia
* Corresponding Author: Ahed Abugabah. Email:
Computer Systems Science and Engineering 2022, 41(3), 997-1008. https://doi.org/10.32604/csse.2022.021438
Received 03 July 2021; Accepted 07 August 2021; Issue published 10 November 2021
Abstract
The outbreak of the novel coronavirus has spread worldwide, and millions of people are being infected. Image or detection classification is one of the first application areas of deep learning, which has a significant contribution to medical image analysis. In classification detection, one or more images (detection) are usually used as input, and diagnostic variables (such as whether there is a disease) are used as output. The novel coronavirus has spread across the world, infecting millions of people. Early-stage detection of critical cases of COVID-19 is essential. X-ray scans are used in clinical studies to diagnose COVID-19 and Pneumonia early. For extracting the discriminative features through these modalities, deep convolutional neural networks (CNNs) are used. A siamese convolutional neural network model (COVID-3D-SCNN) is proposed in this study for the automated detection of COVID-19 by utilizing X-ray scans. To extract the useful features, we used three consecutive models working in parallel in the proposed approach. We acquired 575 COVID-19, 1200 non-COVID, and 1400 pneumonia images, which are publicly available. In our framework, augmentation is used to enlarge the dataset. The findings suggest that the proposed method outperforms the results of comparative studies in terms of accuracy 96.70%, specificity 95.55%, and sensitivity 96.62% over (COVID-19 vs. non-COVID19 vs. Pneumonia).Keywords
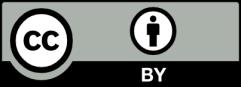