Open Access
ARTICLE
Stochastic Gradient Boosting Model for Twitter Spam Detection
1 Information Technology, Sri Venkateswara College of Engineering, Sriperumbudur, 602117, India
2 Electronics & Communication Engineering, Sri Venkateswara College of Engineering, Sriperumbudur, 602117, India
* Corresponding Author: K. Kiruthika Devi. Email:
Computer Systems Science and Engineering 2022, 41(2), 849-859. https://doi.org/10.32604/csse.2022.020836
Received 10 June 2021; Accepted 23 July 2021; Issue published 25 October 2021
Abstract
In today’s world of connectivity there is a huge amount of data than we could imagine. The number of network users are increasing day by day and there are large number of social networks which keeps the users connected all the time. These social networks give the complete independence to the user to post the data either political, commercial or entertainment value. Some data may be sensitive and have a greater impact on the society as a result. The trustworthiness of data is important when it comes to public social networking sites like facebook and twitter. Due to the large user base and its openness there is a huge possibility to spread spam messages in this network. Spam detection is a technique to identify and mark data as a false data value. There are lot of machine learning approaches proposed to detect spam in social networks. The efficiency of any spam detection algorithm is determined by its cost factor and accuracy. Aiming to improve the detection of spam in the social networks this study proposes using statistical based features that are modelled through the supervised boosting approach called Stochastic gradient boosting to evaluate the twitter data sets in the English language. The performance of the proposed model is evaluated using simulation results.
Keywords
Cite This Article
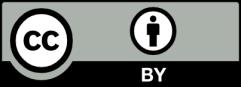
This work is licensed under a Creative Commons Attribution 4.0 International License , which permits unrestricted use, distribution, and reproduction in any medium, provided the original work is properly cited.