Open Access
ARTICLE
Brain Image Classification Using Time Frequency Extraction with Histogram Intensity Similarity
1 Department of Computer Technology-UG, Kongu Engineering College, Erode, India
2 Department of Computer Science, CHRIST (Deemed to be University), Bengaluru, India
3 Department of Computer Science and Engineering, CHRIST (Deemed to be University), Bengaluru, India
* Corresponding Author: Thangavel Renukadevi. Email:
Computer Systems Science and Engineering 2022, 41(2), 645-460. https://doi.org/10.32604/csse.2022.020810
Received 09 June 2021; Accepted 22 July 2021; Issue published 25 October 2021
Abstract
Brain medical image classification is an essential procedure in Computer-Aided Diagnosis (CAD) systems. Conventional methods depend specifically on the local or global features. Several fusion methods have also been developed, most of which are problem-distinct and have shown to be highly favorable in medical images. However, intensity-specific images are not extracted. The recent deep learning methods ensure an efficient means to design an end-to-end model that produces final classification accuracy with brain medical images, compromising normalization. To solve these classification problems, in this paper, Histogram and Time-frequency Differential Deep (HTF-DD) method for medical image classification using Brain Magnetic Resonance Image (MRI) is presented. The construction of the proposed method involves the following steps. First, a deep Convolutional Neural Network (CNN) is trained as a pooled feature mapping in a supervised manner and the result that it obtains are standardized intensified pre-processed features for extraction. Second, a set of time-frequency features are extracted based on time signal and frequency signal of medical images to obtain time-frequency maps. Finally, an efficient model that is based on Differential Deep Learning is designed for obtaining different classes. The proposed model is evaluated using National Biomedical Imaging Archive (NBIA) images and validation of computational time, computational overhead and classification accuracy for varied Brain MRI has been done.Keywords
Cite This Article
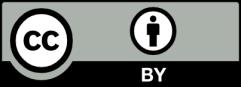
This work is licensed under a Creative Commons Attribution 4.0 International License , which permits unrestricted use, distribution, and reproduction in any medium, provided the original work is properly cited.