Open Access
ARTICLE
Insider Attack Detection Using Deep Belief Neural Network in Cloud Computing
1 School of Computing, E.G.S. Pillay Engineering College, Nagapattinam, 611002, Tamilnadu, India
2 Department of Software Systems, School of Computer Science and Engineering (SCOPE), Vellore Institute of Technology University, Vellore, 632014, Tamilnadu, India
3 Department of Computer Science, CHRIST(Deemed to be University), Bengaluru, 560029, India
4 Department of Information Security, School of Computer Science and Engineering (SCOPE), Vellore Institute of Technology University, Vellore, 632014, Tamilnadu, India
5 Department of Computer Science and Engineering, Kongu Engineering College, Erode, 638060, Tamilnadu, India
* Corresponding Author: A. S. Anakath. Email:
Computer Systems Science and Engineering 2022, 41(2), 479-492. https://doi.org/10.32604/csse.2022.019940
Received 02 May 2021; Accepted 17 June 2021; Issue published 25 October 2021
Abstract
Cloud computing is a high network infrastructure where users, owners, third users, authorized users, and customers can access and store their information quickly. The use of cloud computing has realized the rapid increase of information in every field and the need for a centralized location for processing efficiently. This cloud is nowadays highly affected by internal threats of the user. Sensitive applications such as banking, hospital, and business are more likely affected by real user threats. An intruder is presented as a user and set as a member of the network. After becoming an insider in the network, they will try to attack or steal sensitive data during information sharing or conversation. The major issue in today's technological development is identifying the insider threat in the cloud network. When data are lost, compromising cloud users is difficult. Privacy and security are not ensured, and then, the usage of the cloud is not trusted. Several solutions are available for the external security of the cloud network. However, insider or internal threats need to be addressed. In this research work, we focus on a solution for identifying an insider attack using the artificial intelligence technique. An insider attack is possible by using nodes of weak users’ systems. They will log in using a weak user id, connect to a network, and pretend to be a trusted node. Then, they can easily attack and hack information as an insider, and identifying them is very difficult. These types of attacks need intelligent solutions. A machine learning approach is widely used for security issues. To date, the existing lags can classify the attackers accurately. This information hijacking process is very absurd, which motivates young researchers to provide a solution for internal threats. In our proposed work, we track the attackers using a user interaction behavior pattern and deep learning technique. The usage of mouse movements and clicks and keystrokes of the real user is stored in a database. The deep belief neural network is designed using a restricted Boltzmann machine (RBM) so that the layer of RBM communicates with the previous and subsequent layers. The result is evaluated using a Cooja simulator based on the cloud environment. The accuracy and F-measure are highly improved compared with when using the existing long short-term memory and support vector machine.Keywords
Cite This Article
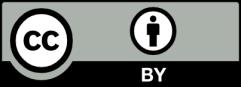
This work is licensed under a Creative Commons Attribution 4.0 International License , which permits unrestricted use, distribution, and reproduction in any medium, provided the original work is properly cited.