Open Access
ARTICLE
Measuring Mental Workload Using ERPs Based on FIR, ICA, and MARA
1 Business School, Shenyang University, Shenyang, 110044, China
2 School of Economics and Management, Anhui Polytechnic University, Wuhu, 241000, China
3 Center for Science Communication and Achievement Transformation, National Natural Science Foundation of China, Beijing, 100085, China
4 School of Industrial Engineering, Purdue University, West Lafayette, IN 47906, USA
* Corresponding Author: Yi Ding. Email:
Computer Systems Science and Engineering 2022, 41(2), 781-794. https://doi.org/10.32604/csse.2022.016387
Received 31 December 2020; Accepted 20 April 2021; Issue published 25 October 2021
Abstract
Mental workload is considered to be strongly linked to human performance, and the ability to measure it accurately is key for balancing human health and work. In this study, brain signals were elicited by mental arithmetic tasks of varying difficulty to stimulate different levels of mental workload. In addition, a finite impulse response (FIR) filter, independent component analysis (ICA), and multiple artifact rejection algorithms (MARAs) were used to filter event-related potentials (ERPs). Then, the data consisting of ERPs, subjective ratings of mental workload, and task performance, were analyzed through the use of variance and Spearman’s correlation during a simulated computer task. We found that participants responded faster and performed better in the easy task condition, followed by the medium and high-difficulty conditions, which verifies the validity of the ERP filtering. Moreover, larger P2 and P3 waveforms were evoked as the task difficulty increased, and a higher task difficulty elicited a more enhanced N300. Correlation analysis revealed a negative relationship between the amplitude of P3 and the subjective ratings, and a positive relationship between the P3 amplitude and accuracy. The results presented in this paper demonstrate that a combination of FIR, ICA, and MARA methods can filter ERPs in the non-invasive real-time measurement of workload. Additionally, frontocentral P2, N3, and parietal P3 components showed differences between genders. The proposed measurement of mental workload can be useful for real-time identification of mental states and can be applied to human–computer interaction in the future.Keywords
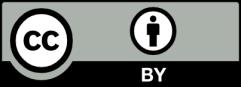