Open Access
ARTICLE
Adaptive Scheme for Crowd Counting Using off-the-Shelf Wireless Routers
1 School of Computer and Software, Nanjing University of Information Science & Technology, Nanjing, 210044, China
2 Engineering Research Center of Digital Forensics, Ministry of Education, Nanjing University of Information Science and Technology, Nanjing, 210044, China
3 School of Engineering & Technology, University of Washington, Tacoma, WA 98402, USA
4 China General Nuclear Power Group, Nanjing, 210028, China
5 Jiangsu Province Hospital of Chinese Medicine, Affiliated Hospital of Nanjing University of Chinese Medicine, Nanjing, 210029, China
* Corresponding Author: Fei Qian. Email:
Computer Systems Science and Engineering 2022, 41(1), 255-269. https://doi.org/10.32604/csse.2022.020590
Received 29 May 2021; Accepted 30 June 2021; Issue published 08 October 2021
Abstract
Since the outbreak of the world-wide novel coronavirus pandemic, crowd counting in public areas, such as in shopping centers and in commercial streets, has gained popularity among public health administrations for preventing the crowds from gathering. In this paper, we propose a novel adaptive method for crowd counting based on Wi-Fi channel state information (CSI) by using common commercial wireless routers. Compared with previous researches on device-free crowd counting, our proposed method is more adaptive to the change of environment and can achieve high accuracy of crowd count estimation. Because the distance between access point (AP) and monitor point (MP) is typically non-fixed in real-world applications, the strength of received signals varies and makes the traditional amplitude-related models to perform poorly in different environments. In order to achieve adaptivity of the crowd count estimation model, we used convolutional neural network (ConvNet) to extract features from correlation coefficient matrix of subcarriers which are insensitive to the change of received signal strength. We conducted experiments in university classroom settings and our model achieved an overall accuracy of 97.79% in estimating a variable number of participants.Keywords
Cite This Article
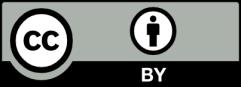
This work is licensed under a Creative Commons Attribution 4.0 International License , which permits unrestricted use, distribution, and reproduction in any medium, provided the original work is properly cited.