Open Access
ARTICLE
Phishing Websites Detection by Using Optimized Stacking Ensemble Model
1 College of Computer Science and Engineering, University of Ha'il, Ha'il, 81481, KSA
2 College of Computer Sciences and Engineering, Hodeidah University, Hodeidah, 967, Yemen
3 College of Computer Science and Engineering, Taibah University, Al-Madinah, 42353, KSA
4 Nottingham Trent University, Mansfield, NG18 5BH, United Kingdom
* Corresponding Author: Badiea Abdulkarem Mohammed. Email:
Computer Systems Science and Engineering 2022, 41(1), 109-125. https://doi.org/10.32604/csse.2022.020414
Received 22 May 2021; Accepted 23 June 2021; Issue published 08 October 2021
Abstract
Phishing attacks are security attacks that do not affect only individuals’ or organizations’ websites but may affect Internet of Things (IoT) devices and networks. IoT environment is an exposed environment for such attacks. Attackers may use thingbots software for the dispersal of hidden junk emails that are not noticed by users. Machine and deep learning and other methods were used to design detection methods for these attacks. However, there is still a need to enhance detection accuracy. Optimization of an ensemble classification method for phishing website (PW) detection is proposed in this study. A Genetic Algorithm (GA) was used for the proposed method optimization by tuning several ensemble Machine Learning (ML) methods parameters, including Random Forest (RF), AdaBoost (AB), XGBoost (XGB), Bagging (BA), GradientBoost (GB), and LightGBM (LGBM). These were accomplished by ranking the optimized classifiers to pick out the best classifiers as a base for the proposed method. A PW dataset that is made up of 4898 PWs and 6157 legitimate websites (LWs) was used for this study's experiments. As a result, detection accuracy was enhanced and reached 97.16 percent.Keywords
Cite This Article
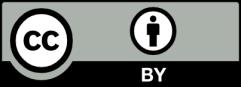
This work is licensed under a Creative Commons Attribution 4.0 International License , which permits unrestricted use, distribution, and reproduction in any medium, provided the original work is properly cited.