Open Access
ARTICLE
Gaussian Kernel Based SVR Model for Short-Term Photovoltaic MPP Power Prediction
Department of Electrical and Electronics Engineering, Bilecik Seyh Edebali University, Bilecik, 11000, Turkey
* Corresponding Author: Yasemin Onal. Email:
Computer Systems Science and Engineering 2022, 41(1), 141-156. https://doi.org/10.32604/csse.2022.020367
Received 20 May 2021; Accepted 21 June 2021; Issue published 08 October 2021
Abstract
Predicting the power obtained at the output of the photovoltaic (PV) system is fundamental for the optimum use of the PV system. However, it varies at different times of the day depending on intermittent and nonlinear environmental conditions including solar irradiation, temperature and the wind speed, Short-term power prediction is vital in PV systems to reconcile generation and demand in terms of the cost and capacity of the reserve. In this study, a Gaussian kernel based Support Vector Regression (SVR) prediction model using multiple input variables is proposed for estimating the maximum power obtained from using perturb observation method in the different irradiation and the different temperatures for a short-term in the DC-DC boost converter at the PV system. The performance of the kernel-based prediction model depends on the availability of a suitable kernel function that matches the learning objective, since an unsuitable kernel function or hyper parameter tuning results in significantly poor performance. In this study for the first time in the literature both maximum power is obtained at maximum power point and short-term maximum power estimation is made. While evaluating the performance of the suggested model, the PV power data simulated at variable irradiations and variable temperatures for one day in the PV system simulated in MATLAB were used. The maximum power obtained from the simulated system at maximum irradiance was 852.6 W. The accuracy and the performance evaluation of suggested forecasting model were identified utilizing the computing error statistics such as root mean square error (RMSE) and mean square error (MSE) values. MSE and RMSE rates which obtained were 4.5566 * 10−04 and 0.0213 using ANN model. MSE and RMSE rates which obtained were 13.0000 * 10−04 and 0.0362 using SWD-FFNN model. Using SVR model, 1.1548 * 10−05 MSE and 0.0034 RMSE rates were obtained. In the short-term maximum power prediction, SVR gave higher prediction performance according to ANN and SWD-FFNN.Keywords
Cite This Article
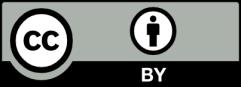
This work is licensed under a Creative Commons Attribution 4.0 International License , which permits unrestricted use, distribution, and reproduction in any medium, provided the original work is properly cited.