Open Access
ARTICLE
Ensemble Variable Selection for Naive Bayes to Improve Customer Behaviour Analysis
1 Anna University, Chennai, 600025, India
2 Department of Computer Science and Engineering, Sri Krishna College of Engineering and Technology, Coimbatore, 641008, India
* Corresponding Author: R. Siva Subramanian. Email:
Computer Systems Science and Engineering 2022, 41(1), 339-355. https://doi.org/10.32604/csse.2022.020043
Received 07 May 2021; Accepted 20 June 2021; Issue published 08 October 2021
Abstract
Executing customer analysis in a systemic way is one of the possible solutions for each enterprise to understand the behavior of consumer patterns in an efficient and in-depth manner. Further investigation of customer patterns helps the firm to develop efficient decisions and in turn, helps to optimize the enterprise’s business and maximizes consumer satisfaction correspondingly. To conduct an effective assessment about the customers, Naive Bayes(also called Simple Bayes), a machine learning model is utilized. However, the efficacious of the simple Bayes model is utterly relying on the consumer data used, and the existence of uncertain and redundant attributes in the consumer data enables the simple Bayes model to attain the worst prediction in consumer data because of its presumption regarding the attributes applied. However, in practice, the NB premise is not true in consumer data, and the analysis of these redundant attributes enables simple Bayes model to get poor prediction results. In this work, an ensemble attribute selection methodology is performed to overcome the problem with consumer data and to pick a steady uncorrelated attribute set to model with the NB classifier. In ensemble variable selection, two different strategies are applied: one is based upon data perturbation (or homogeneous ensemble, same feature selector is applied to a different subsamples derived from the same learning set) and the other one is based upon function perturbation (or heterogeneous ensemble different feature selector is utilized to the same learning set). Furthermore, the feature set captured from both ensemble strategies is applied to NB individually and the outcome obtained is computed. Finally, the experimental outcomes show that the proposed ensemble strategies perform efficiently in choosing a steady attribute set and increasing NB classification performance efficiently.Keywords
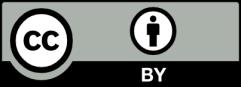