Open Access
ARTICLE
A Secure IoT-Cloud Based Healthcare System for Disease Classification Using Neural Network
Department of Computer Science and Engineering, R.M.D. Engineering College, Kavaraipettai, 601206, Tamilnadu, India
* Corresponding Author: M. Vedaraj. Email:
Computer Systems Science and Engineering 2022, 41(1), 95-108. https://doi.org/10.32604/csse.2022.019976
Received 04 May 2021; Accepted 18 June 2021; Issue published 08 October 2021
Abstract
The integration of the Internet of Things (IoT) and cloud computing is the most popular growing technology in the IT world. IoT integrated cloud computing technology can be used in smart cities, health care, smart homes, environmental monitoring, etc. In recent days, IoT integrated cloud can be used in the health care system for remote patient care, emergency care, disease prediction, pharmacy management, etc. but, still, security of patient data and disease prediction accuracy is a major concern. Numerous machine learning approaches were used for effective early disease prediction. However, machine learning takes more time and less performance while classification. In this research work, the Attribute based Searchable Honey Encryption with Functional Neural Network (ABSHE-FNN) framework is proposed to analyze the disease and provide stronger security in IoT-cloud healthcare data. In this work, the Cardiovascular Disease and Pima Indians diabetes dataset are used for heart and diabetic disease classification. Initially, means-mode normalization removes the noise and normalizes the IoT data, which helps to enhance the quality of data. Rectified Linear Unit (RLU) was applied to adjust the feature weight to reduce the training cost and error classification. This proposed ABSHE-FNN technique provides better security and achieves 92.79% disease classification accuracy compared to existing techniques.Keywords
Cite This Article
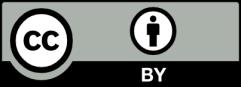