Open Access
ARTICLE
Integrated Approach to Detect Cyberbullying Text: Mobile Device Forensics Data
1 Department of Computer Science and Engineering, Saveetha Engineering College, Chennai, 602105, India
2 Department of Computer Science and Engineering, School of Computing, SRM Institute of Science and Technology, Chengalpattu, 603203, India
3 Department of Information Technology, Saveetha Engineering College, Chennai, 602105, India
* Corresponding Author: G. Maria Jones. Email:
Computer Systems Science and Engineering 2022, 40(3), 963-978. https://doi.org/10.32604/csse.2022.019483
Received 14 April 2021; Accepted 23 May 2021; Issue published 24 September 2021
Abstract
Mobile devices and social networks provide communication opportunities among the young generation, which increases vulnerability and cybercrimes activities. A recent survey reports that cyberbullying and cyberstalking constitute a developing issue among youngsters. This paper focuses on cyberbullying detection in mobile phone text by retrieving with the help of an oxygen forensics toolkit. We describe the data collection using forensics technique and a corpus of suspicious activities like cyberbullying annotation from mobile phones and carry out a sequence of binary classification experiments to determine cyberbullying detection. We use forensics techniques, Machine Learning (ML), and Deep Learning (DL) algorithms to exploit suspicious patterns to help the forensics investigation where every evidence contributes to the case. Experiments on a real-time dataset reveal better results for the detection of cyberbullying content. The Random Forest in ML approach produces 87% of accuracy without SMOTE technique, whereas the value of F1Score produces a good result with SMOTE technique. The LSTM has 92% of validation accuracy in the DL algorithm compared with Dense and BiLSTM algorithms.Keywords
Cite This Article
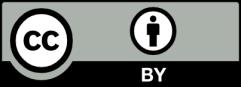