Open Access
ARTICLE
IoT Wireless Intrusion Detection and Network Traffic Analysis
1 University Tunku Abdul Rahman, Kampar, 31900, Malaysia
2 School of Computer Science and Engineering (SCE), Taylor’s University, Selangor, Malaysia
3 Department of Information Systems, College of Computer and Information Sciences, Jouf University, Al-Jouf, Saudi Arabia
* Corresponding Author: NZ Jhanjhi. Email:
Computer Systems Science and Engineering 2022, 40(3), 865-879. https://doi.org/10.32604/csse.2022.018801
Received 22 March 2021; Accepted 05 May 2021; Issue published 24 September 2021
Abstract
Enhancement in wireless networks had given users the ability to use the Internet without a physical connection to the router. Almost every Internet of Things (IoT) devices such as smartphones, drones, and cameras use wireless technology (Infrared, Bluetooth, IrDA, IEEE 802.11, etc.) to establish multiple inter-device connections simultaneously. With the flexibility of the wireless network, one can set up numerous ad-hoc networks on-demand, connecting hundreds to thousands of users, increasing productivity and profitability significantly. However, the number of network attacks in wireless networks that exploit such flexibilities in setting and tearing down networks has become very alarming. Perpetrators can launch attacks since there is no first line of defense in an ad hoc network setup besides the standard IEEE802.11 WPA2 authentication. One feasible countermeasure is to deploy intrusion detection systems at the edge of these ad hoc networks (Network-based IDS) or at the node level (Host-based IDS). The challenge here is that there is no readily available benchmark data available for IoT network traffic. Creating this benchmark data is very tedious as IoT can work on multiple platforms and networks, and crafting and labelling such dataset is very labor-intensive. This research aims to study the characteristics of existing datasets available such as KDD-Cup and NSL-KDD, and their suitability for wireless IDS implementation. We hypothesize that network features are parametrically different depending on the types of network and assigning weight dynamically to these features can potentially improve the subsequent threat classifications. This paper analyses packet and flow features for the data packet captured on a wireless network rather than a wired network. Combining domain heuristcs and early classification results, the paper had identified 19 header fields exclusive to wireless network that contain high information gain to be used as ML features in Wireless IDS.Keywords
Cite This Article
Citations
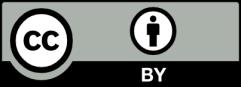
This work is licensed under a Creative Commons Attribution 4.0 International License , which permits unrestricted use, distribution, and reproduction in any medium, provided the original work is properly cited.