Open Access
ARTICLE
Classification and Diagnosis of Lymphoma’s Histopathological Images Using Transfer Learning
College of Computers and Information Technology, Computer Engineering Department, Taif University, Taif, Kingdom of Saudi Arabia
* Corresponding Author: Schahrazad Soltane. Email:
Computer Systems Science and Engineering 2022, 40(2), 629-644. https://doi.org/10.32604/csse.2022.019333
Received 10 April 2021; Accepted 14 May 2021; Issue published 09 September 2021
Abstract
Current cancer diagnosis procedure requires expert knowledge and is time-consuming, which raises the need to build an accurate diagnosis support system for lymphoma identification and classification. Many studies have shown promising results using Machine Learning and, recently, Deep Learning to detect malignancy in cancer cells. However, the diversity and complexity of the morphological structure of lymphoma make it a challenging classification problem. In literature, many attempts were made to classify up to four simple types of lymphoma. This paper presents an approach using a reliable model capable of diagnosing seven different categories of rare and aggressive lymphoma. These Lymphoma types are Classical Hodgkin Lymphoma, Nodular Lymphoma Predominant, Burkitt Lymphoma, Follicular Lymphoma, Mantle Lymphoma, Large B-Cell Lymphoma, and T-Cell Lymphoma. Our proposed approach uses Residual Neural Networks, ResNet50, with a Transfer Learning for lymphoma’s detection and classification. The model used results are validated according to the performance evaluation metrics: Accuracy, precision, recall, F-score, and kappa score for the seven multi-classes. Our algorithms are tested, and the results are validated on 323 images of 224 × 224 pixels resolution. The results are promising and show that our used model can classify and predict the correct lymphoma subtype with an accuracy of 91.6%.Keywords
Cite This Article
Citations
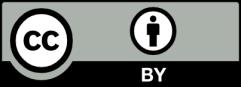
This work is licensed under a Creative Commons Attribution 4.0 International License , which permits unrestricted use, distribution, and reproduction in any medium, provided the original work is properly cited.