Open Access
ARTICLE
Design of Neural Network Based Wind Speed Prediction Model Using GWO
1 Department of Computer Science and Engineering, Sri Ramakrishna Engineering College, Coimbatore, 641022, India
2 Department of Computer Science and Engineering, PSG Institute of Technology and Applied Research, Coimbatore, 641062, India
* Corresponding Author: R. Kingsy Grace. Email:
Computer Systems Science and Engineering 2022, 40(2), 593-606. https://doi.org/10.32604/csse.2022.019240
Received 07 April 2021; Accepted 14 May 2021; Issue published 09 September 2021
Abstract
The prediction of wind speed is imperative nowadays due to the increased and effective generation of wind power. Wind power is the clean, free and conservative renewable energy. It is necessary to predict the wind speed, to implement wind power generation. This paper proposes a new model, named WT-GWO-BPNN, by integrating Wavelet Transform (WT), Back Propagation Neural Network (BPNN) and Grey Wolf Optimization (GWO). The wavelet transform is adopted to decompose the original time series data (wind speed) into approximation and detailed band. GWO – BPNN is applied to predict the wind speed. GWO is used to optimize the parameters of back propagation neural network and to improve the convergence state. This work uses wind power data of six months with 25, 086 data points to test and verify the performance of the proposed model. The proposed work, WT-GWO-BPNN, predicts the wind speed using a three-step procedure and provides better results. Mean Absolute Error (MAE), Mean Squared Error (MSE), Mean absolute percentage error (MAPE) and Root mean squared error (RMSE) are calculated to validate the performance of the proposed model. Experimental results demonstrate that the proposed model has better performance when compared to other methods in the literature.Keywords
Cite This Article
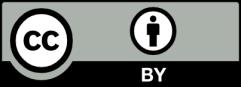
This work is licensed under a Creative Commons Attribution 4.0 International License , which permits unrestricted use, distribution, and reproduction in any medium, provided the original work is properly cited.