Open Access
ARTICLE
An improved CRNN for Vietnamese Identity Card Information Recognition
1 Information Science Faculty, Sai Gon University, HCM City, 700000, Vietnam
2 Faculty of Electrical & Electronics Engineering, University of Technology, HCM City, 700000, Vietnam
* Corresponding Author: Pham The Bao. Email:
Computer Systems Science and Engineering 2022, 40(2), 539-555. https://doi.org/10.32604/csse.2022.019064
Received 31 March 2021; Accepted 10 May 2021; Issue published 09 September 2021
Abstract
This paper proposes an enhancement of an automatic text recognition system for extracting information from the front side of the Vietnamese citizen identity (CID) card. First, we apply Mask-RCNN to segment and align the CID card from the background. Next, we present two approaches to detect the CID card’s text lines using traditional image processing techniques compared to the EAST detector. Finally, we introduce a new end-to-end Convolutional Recurrent Neural Network (CRNN) model based on a combination of Connectionist Temporal Classification (CTC) and attention mechanism for Vietnamese text recognition by jointly train the CTC and attention objective functions together. The length of the CTC’s output label sequence is applied to the attention-based decoder prediction to make the final label sequence. This process helps to decrease irregular alignments and speed up the label sequence estimation during training and inference, instead of only relying on a data-driven attention-based encoder-decoder to estimate the label sequence in long sentences. We may directly learn the proposed model from a sequence of words without detailed annotations. We evaluate the proposed system using a real collected Vietnamese CID card dataset and find that our method provides a 4.28% in WER and outperforms the common techniques.Keywords
Cite This Article
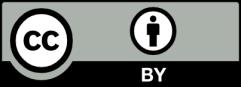