Open Access
ARTICLE
A New Random Forest Applied to Heavy Metal Risk Assessment
1 Wuhan Polytechnic University, Department of Mathematics and Computer Science, Wuhan, 430023, China
2 Northeastern State University, Department of Mathematics and Computer Science, Tahlequah, OK, 74464, USA
* Corresponding Author: Cong Zhang. Email:
Computer Systems Science and Engineering 2022, 40(1), 207-221. https://doi.org/10.32604/csse.2022.018301
Received 04 March 2021; Accepted 30 April 2021; Issue published 26 August 2021
Abstract
As soil heavy metal pollution is increasing year by year, the risk assessment of soil heavy metal pollution is gradually gaining attention. Soil heavy metal datasets are usually imbalanced datasets in which most of the samples are safe samples that are not contaminated with heavy metals. Random Forest (RF) has strong generalization ability and is not easy to overfit. In this paper, we improve the Bagging algorithm and simple voting method of RF. A W-RF algorithm based on adaptive Bagging and weighted voting is proposed to improve the classification performance of RF on imbalanced datasets. Adaptive Bagging enables trees in RF to learn information from the positive samples, and weighted voting method enables trees with superior performance to have higher voting weights. Experiments were conducted using G-mean, recall and F1-score to set weights, and the results obtained were better than RF. Risk assessment experiments were conducted using W-RF on the heavy metal dataset from agricultural fields around Wuhan. The experimental results show that the RW-RF algorithm, which use recall to calculate the classifier weights, has the best classification performance. At the end of this paper, we optimized the hyperparameters of the RW-RF algorithm by a Bayesian optimization algorithm. We use G-mean as the objective function to obtain the optimal hyperparameter combination within the number of iterations.Keywords
Cite This Article
Citations
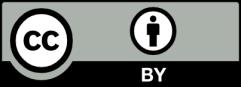
This work is licensed under a Creative Commons Attribution 4.0 International License , which permits unrestricted use, distribution, and reproduction in any medium, provided the original work is properly cited.